- R&D theme № 0123U100866: «Methods and models for identifying object states in medical decision-making tasks».
Supervisor: PhD in Technical Science, Doctor of Biological Sciences, Prof. Ievgen Nastenko. Duration: 2023-2028.
The aim of the project is to develop and promote new approaches based on machine learning to assess the state of biological entities and provide support for medical decision-making. As part of the project, collaboration is ongoing with the Biometric Technology Laboratory of the University of Calgary to create a stress monitoring system based on physiological indicators collected by wearable electronic devices.
Potential application areas: systems for monitoring the condition of athletes, emergency responders, military personnel, patients, and psychological prevention in the general population.
- R&D theme № 117U006934: «Methods and models for identifying states of biological entities».
- Supervisor: PhD in Technical Science, Doctor of Biological Sciences, Prof. Ievgen Nastenko. Duration: 2017-2022.
The R&D topic has been implemented through a series of projects aimed at developing new efficient approaches to address pressing challenges in automated medical image diagnostics, differentiation of diseases with similar clinical manifestations, and patient state evaluation. Project developments are based on studies and novel results in the field of texture analysis, classifier construction algorithms in the Random Forest class, modeling of hybrid classifiers based on convolutional networks and self-organized forests, and class-oriented selection technologies in classification tasks with a very large number of features.
Project 1.
Classification of normal-pathology state in diffuse diseases using ultrasonic images of the liver
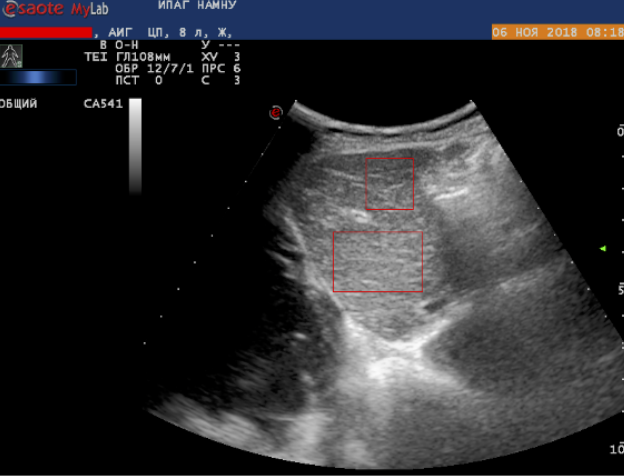
The system determines a preliminary diagnosis of “normal-pathology” for a range of diffuse liver diseases, the most common of which are hepatic steatosis, hepatitis, fibrosis, and cirrhosis of the liver. For a data set with hepatitis B and C, autoimmune hepatitis, Wilson’s disease, steatosis, and liver cirrhosis, the authors developed a classifier: an enhanced version of the random forest.
Classification results were obtained at the level of the best global works for similar tasks. Diagnostic accuracy ranged from 90.3 to 100 percent on the test sample, depending on the type of ultrasonic investigation sensor. The results have been approved at the State Institute of Nuclear Medicine and Radiation Diagnostics in the form of a developed web-based decision support system. Studies continue with the aim of detailing the diagnostic conclusion: determining the degree of liver fibrosis.
The work is protected by copyrights, articles indexed in the Scopus scientific metric database, and sections of the Springer publishing house monograph.
Copyright certificates:
-Patent of Ukraine for a utility model No. 139916 “Method of diagnosing diffuse liver diseases in children“. Inventors: Dykan I.M., Nastenko E.A., Maksimenko V.B., Pavlov V.A., Kruglyi V.V., Tarasyuk B.A., Berezenco V.S., Solodushchenko V.V., patent holders – State Institution “Institute of Pediatrics, Obstetrics and Gynecology named after Academician O.M.Lukyanova of the NAMS of Ukraine” and State Institution “Institute of Nuclear Medicine and Radiation Diagnostics of the NAMS of Ukraine”
– Property rights to the scientific work: “Decision support system for diagnosing fibrous liver changes in children” by Dykan Irina, Maksimenko Vitaliy, Nastenko Ievgen, Tarasyuk Andriy, Pavlov Volodymyr, Kruglyi Vladyslav, Nosovets Olena, Babenko Vitaliy, Goncharuk Maksim, Berezenco Valentina, Solodushchenko Volodymyr. State Property Institute of Ukraine. Registration number 6461 dated December 29, 2021.
– Property rights to the scientific work: “System for detecting chronic diffuse liver diseases” by Dykan Irina, Nastenko Ievgen, Pavlov Volodymyr, Tarasyuk Andriy, Babenko Vitaliy, Kruglyi Vladyslav, Solodushchenko Volodymyr, Goncharuk Maksim, Averianova Olha. State Property Institute of Ukraine. Registration number 6659 dated January 13, 2023
Links to Scopus Articles and Springer’s Publishing House Section:
– Ievgen Nastenko, Olena Nosovets, Vitalii Babenko, Marina Dyba, Vitalii Maksymenko, Boris Tarasiuk, Vladyslav Kruhlyi, Vitalij Umanets, Irina Dykan, Volodymyr Pavlov, Volodymyr Soloduschenko / Liver Pathological States Identification in Diffuse Diseases with Self-Organization Models Based on Ultrasound Images Texture Features. // Proceedings of the XV IEEE International Conference CSIT-20& International Workshop on Inductive Modeling. Zbarazh Castle, UKRAINE, 23-26 September, 2020 – 314 p. / P. 21-26, ISSN 2194-5357 ISSN 2194-5365 https://doi.org/10.1007/978-3-030-63270-0, (Scopus)
– Nastenko I., Maksymenko V., Galkin A., Pavlov V., Nosovets O., Dykan I., Tarasiuk B., Babenko V., Umanets V., Petrunina O. Klymenko D., (2021) Liver Pathological States Identification with Self-organization Models Based on Ultrasound Images Texture Features. In: Shakhovska N., Medykovskyy M.O. (eds) Advances in Intelligent Systems and Computing V. CSIT 2020. Advances in Intelligent Systems and Computing, vol 1293. Springer, Cham. https://doi.org/10.1007/978-3-030-63270-0_26
– Hrishko D., Trofymenko O., Nosovets O., Bovsunoskaja K., Dykan I., Tarasiuk B. Pavlov V., Nastenko Ie. Optimal Complexity Structures of Ultrasound Image Models in Diagnostic Decision-Making System// Proceedings of the XVI IEEE International Conference CSIT-21& International Workshop on Inductive Modeling. Lviv, UKRAINE, 23-26 September, 2021 P. 390-393. DOI: 10.1109/CSIT52700.2021.9648686, ISBN Information: Electronic ISBN:978-1-6654-4257-2, Print on Demand(PoD) ISBN:978-1-6654-4258-9,ISSN Information:Electronic ISSN: 2766-3639, Print on Demand(PoD) ISSN: 2766-3655, https://ieeexplore.ieee.org/document/9648686/ (Scopus)
Project-2:
Identification of ischemic heart disease using video data from speckle-tracking echocardiography with the application of dobutamine stress test
The study was conducted at the National Institute of Cardiovascular Surgery named after M. Amosov. The work is unique for decision support systems in ischemic heart disease. The diagnostic classifier developed by the authors showed the best result compared to known statistical algorithms: Logistic Regression, AdaBoost, Random Forest. The achieved classification accuracy ranges from 83.3 to 90.3 percent on the test sample, depending on the shooting position: bi-chamber, four-chamber, longitudinal axis. The research results are protected by articles, including those indexed in the Scopus scientometric database.
Reference to publications:
– Nastenko, I., Maksymenko, V., Potashev, S., Pavlov, V., Babenko, V., Rysin, S., Matviichuk, O., & Lazoryshinets, V. (2021). Random Forest Algorithm Construction for the Diagnosis of Coronary Heart Disease Based on Echocardiography Video Data Streams. Innovative Biosystems and Bioengineering, 5(1), 61–69. https://doi.org/10.20535/ibb.2021.5.1.225794
-Olena Petrunina, Diana Shevaga, Vitalii Babenko, Volodymyr Pavlov, Sergiy Rysin, Ievgen Nastenko Comparative Analysis of Classification Algorithms in the Analysis of Medical Images From Speckle Tracking Echocardiography Video Data. Innovative Biosystems and Bioengineering, 5(3), (2021), 153-166.
– V. A. Smiianov, S. A. Rudenko, S. V. Potashev, S. V. Salo, A. Y. Gavrylyshin, E. V. Levchyshina, L. M. Hrubyak, E. K. Nosovets, E. A. Nastenko, A. V. Rudenko, and V. V. Lazoryshynets, “Speckle tracking dobutamine stress echocardiography diagnostic accuracy in primary coronary arteries disease diagnosis,” Wiadomości Lekarskie, vol. 73, no. 11, pp. 2447–2456, 2020. DOI: 10.36740/WLek202011121 (Scopus)
Project-3
Diagnosis and Classification of Lung Lesion Types (Ground Glass Opacity, Crazy Paving, Consolidation) Based on Computer Tomography Images in Covid-19 Patients
The research was provided by the state institution “National Institute of Phthisiatry and Pulmonology named after F.G. Yanovsky of the National Academy of Medical Sciences of Ukraine”. For lesion diagnosis, a hybrid classification system was developed based on a convolutional neural network and a self-organized forest. The classification results of lung lesion types in Covid-19 patients achieved are on par with the best global research outcomes for this task. The achieved classification accuracy level is 95% on the test data sample
The lung lesion diagnosis and classification system for COVID-19 has been implemented at the “National Institute of Phthisiatry and Pulmonology named after F.G. Yanovsky of the National Academy of Medical Sciences of Ukraine”.
Work is ongoing.
The findings are backed by articles indexed in the Scopus bibliographic database.
Reference to publications:
-Davydko O., Hladkyi Y., Linnik M., Nosovets O., Pavlov V., Nastenko Ie. / Hybrid Classifiers Based on CNN, LSOF, GMDH in COVID-19 Pneumonic Lesions Types Classification Task // Proceedings of the XVI IEEE International Conference CSIT-21& International Workshop on Inductive Modeling. Lviv, UKRAINE, 23-26 September, 2021 P. 380-384. DOI: 10.1109/CSIT52700.2021.9648752, ІSBN Information: Electronic ISBN:978-1-6654-4257-2, Print on Demand(PoD) ISBN:978-1-6654-4258-9, ISSN Information:Electronic ISSN: 2766-3639 , Print on Demand(PoD) ISSN: 2766-3655, https://ieeexplore.ieee.org/document/9648752 (Scopus)
-Davydko O., Hladkyi Y., Linnik M., Horodetska O., Pavlov V., Galkin O., Nastenko Ie., Longo L. “A pipeline for the Diagnosis and Classification of Lung Lesions for patients with COVID-19” 2022 IEEE 17th International Conference on Computer Sciences and Information Technologies (CSIT), 2022 https://ieeexplore.ieee.org/document/10000435 (Scopus)
Project-4:
Differentiation of Chemoresistant and Chemosensitive Forms of Tuberculosis Based on Lung Computer Tomography Images
The research was provided by the state institution “National Institute of Phthisiatry and Pulmonology named after F.G. Yanovsky of the National Academy of Medical Sciences of Ukraine”. For the differential diagnosis of chemoresistant tuberculosis, a class-oriented technology for the selection of textural features from lung images was developed, along with new improved classifiers based on convolutional networks and decision tree forests. The diagnostic results achieved are on par with the best global research outcomes for this task
The classification accuracy of the approaches proposed by the authors ranges from 85-95% on the test sample, depending on the proposed approach and the subject of diagnosis (specific areas of interest or the entire lungs highlighted). The results have been implemented at the National Institute of Phthisiatry and Pulmonology named after F.G. Yanovsky of the National Academy of Medical Sciences of Ukraine
Work is ongoing.
The findings are backed by articles indexed in the Scopus bibliographic database.
Reference to publications:
Matviichuk O., Nosovets O., Linnik M., Davydko O., Pavlov V., Nastenko Ie. / Class-Oriented Features Selection Technology in Medical Images Classification Problem on the Example of Distinguishing Between Tuberculosis Sensitive and Resistant Forms// Proceedings of the XVI IEEE International Conference CSIT-21& International Workshop on Inductive Modeling. Lviv, UKRAINE, 23-26 September, 2021 P. 385-389, DOI: 10.1109/CSIT52700.2021.9648747, (Scopus)
-Matviichuk O., Horodetska O., Linnik M., Biloshytska O., Pavlov V. and Nastenko Ie”Positional Approach to the Voting Function Formation of Random Forest Trees as an Example of Solving the Differentiating Tuberculosis Forms Problem” 2022 IEEE 17th International Conference on Computer Sciences and Information Technologies (CSIT), 2022 https://ieeexplore.ieee.org/document/10000450, (Scopus)
Project-5:
New technologies for evaluating functional and compensatory reserves of the cardiovascular system in youth
In collaboration with the Department of Physical Education of Biomedical Engineering Faculty, based on over 3,500 examinations, students’ circulatory system reactions to physical exertion were studied. Risk groups for sudden circulatory disorders were identified, and diagnostic models were constructed. The results have been integrated into the educational process.
Results obtained while working on the R&D theme № 117U006934:
“Methods and models for the identification of states of biological entities” were used in the teaching of disciplines of the first, second, and third levels of higher education, as well as in master’s and postgraduate theses. Involved in the implementation of the topic: postgraduate students – 3, students – 14; defended master’s dissertations – 9; published: 1 textbook, 17 articles in professional publications (Scopus indexed articles – 5), chapters in monographs in foreign publications – 1. Seven master’s degree students co-authored research articles indexed in Scopus and Web of Science databases.
- Project
Blood pressure estimation, according to the contract RD/786/09-2018 with Samsung Electronics Ukraine Company. Project supervisor: Dr. of Biol. Sc., Cand. of Tech. Sc., Ievgen Nastenko
- Project
«Segmentation of the left ventricle on the heart MRI images» according to the contract RD/676/30-0413 з Samsung Electronics Ukraine Company, Project supervisor: Dr. of Biol. Sc., Cand. of Tech. Sc., Ievgen Nastenko
- Project
«Cardiac LV endo contour automatic detection» according to the contract RD/345/21-0114 with Samsung Electronics Ukraine Company, Project supervisor: Dr. of Biol. Sc., Cand. of Tech. Sc., Ievgen Nastenko
- Project
«Development of robust method for automatic detection of anatomical heart features for cardiac analysis»
according to the contract RD/389/20-0614 with Samsung Electronics Ukraine Company, Project supervisor: Dr. of Biol. Sc., Cand. of Tech. Sc., Ievgen Nastenko
- Project EUMLS «Ordinal time series and their application for EEG/ECG signal analysis» Grant number PIRSES-GA-2011-295164-EUMLS (04.2012 – 03.2016) Project coordinator: University of Lübeck (Germany). Project supervisor: Dr. of Med. Sc., Prof. Maksymenko V. B.